Practical AI: the Data Science Hierarchy of Needs
How to make AI a sustainable revenue generator and not just a shiny (and costly) toy
There is a famous principle in psychology called Maslow’s hierarchy of needs that posits humans require basic fundamental needs be met before they are capable of performing higher order tasks. For instance, food, shelter and social needs must be met before someone can make consistent, stable progress on personal growth and new skills. Shortcuts to self-actualization that build on unstable foundations are neither reliable nor will they progress smoothly.
Similarly data science has a hierarchy of needs whereby continuously-learning production AI systems are only possible and stable when built upon a solid foundation of existing capabilities.
Here I describe that hierarchy of needs, its implications for making profitable data science product suites, and some key takeaways for individual businesses and the evolution of AI in markets.
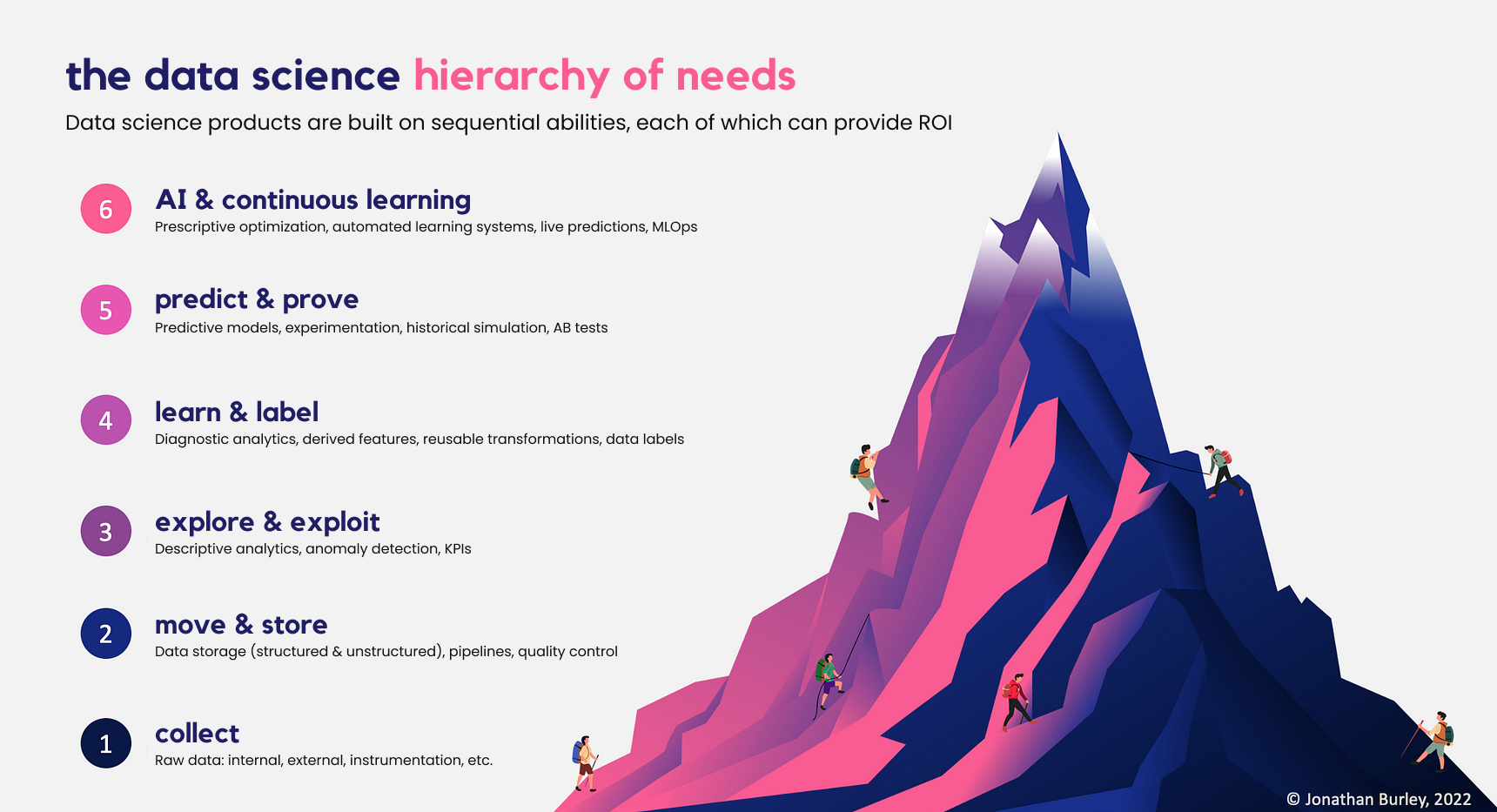
The hierarchy mountain required depends on the product: analysis identifying a process change to improve business may require only tiers 1–3 for a limited amount of data; an AI customer engagement platform requires tiers 1–6 for a large amount of data. Consider this as the product determining where the peak of the mountain is.
At the risk of mixing metaphors, the data science hierarchy isn’t just a mountain you climb, it’s a mountain you build: higher tiers are built on lower tiers; the lower tiers have more mass (time investment) than do higher tiers and the exact nature of the highest point on the mountain determines what needs to be built below for the system to be stable.
After finishing the mountain that supports a single ML product, the question is “What next?”. It is easy to treat the second, third, and fourth product like the first: decide on the product and make our mountainous path there. But that would be a mistake. In a sane business, the data science products will perform similar calculations or use similar data and those similarities are work that should only be done once.
"if AI is a mountain that needs to be climbed then a suite of AI products are a mountain range that shares the same roots rather than independent mountains"
If AI is a mountain that needs to be climbed then a suite of AI products are a mountain range that shares the same roots rather than independent mountains. Considered that way, each new functionality has value for all future uses (not just the current project).
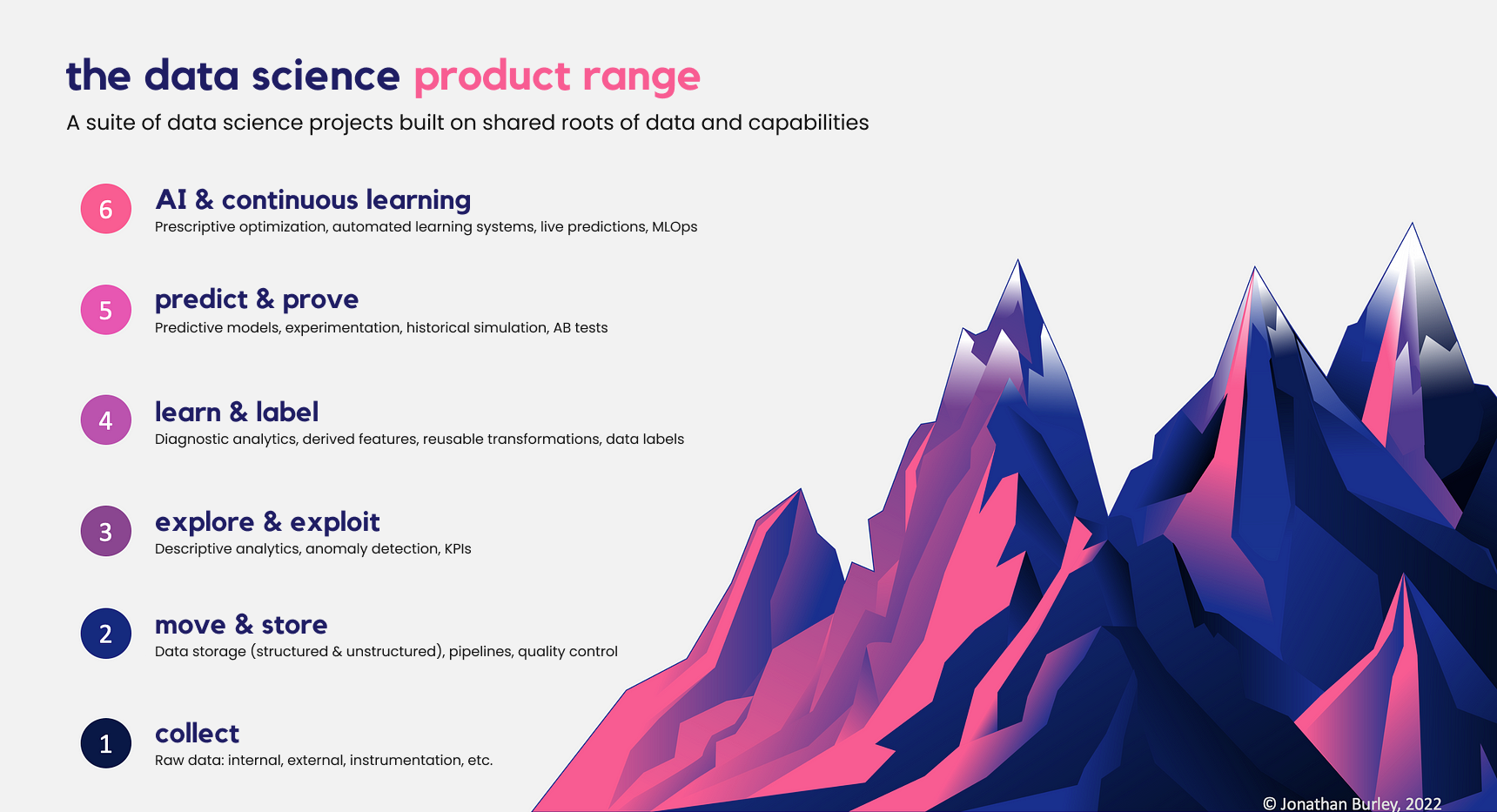
Our best bets on what will be the second-through-fourth products should inform how earlier products are built, or even which ones are built. An accurate net present value of data science investment comes from a holistic overview of all projects and their interdependencies not just the sum of each project independently. In other words, (e.g.) a long-lived data source has net present value from future products, and awareness of such reusable components changes product priorities.
Therefore expanding our view of the hierarchy of needs has important implications for how a data science ecosystem should evolve and how we should be thinking about making data science products.
take homes
Thinking about a data science ecosystem as a mountain range of products creates some core advice for businesses —
Basic advice for all businesses:
- Machine Learning & AI is not the only value in Data Science. Data-based decision making can realize value long before an AI system is built.
- Data and infrastructure systems can (and should) support many products, including some that are neither known nor obvious when the system is built. It is smart to build data systems that are robust to several different use cases and provide clear provenance for all data.
- Data science capabilities require human expertise just as much as databases, documentation, and computational power. You need to retain your key talent over several projects.
- Like any other investment, data science is a balance of upfront costs, probable returns, and how long until those returns are realized. Not all projects should invest equally in reusable infrastructure and data, and data science leadership should have the discipline to make that call.
For a traditional business exploring AI:
- In building the mountain, each phase can make returns that support the initial investment. A self-sustaining data science process is a useful discipline for the CEO to enforce (don’t pay for items with uncertain payoff in the distant future), and the DS leadership (don’t be a cost centre, that is the best way to get eliminated in a belt-tightening exercise).
- With a clear roadmap, costs can be controlled by only hiring staff needed at that time. Hiring many data scientists and MLOps staff several years before there exists the data infrastructure to support their most specialized skills is a poor use of resources.
For an AI pureplay:
- Foundational capabilities are essential to long-term growth. Bootstrapping an AI application on weak datasets and hard-to-scale operational infrastructure is a technical debt that doesn’t just have to be paid off on your first product idea, it will also have to be paid off before making your second idea. (This is not to disparage the value of MVP prototypes — they are the best way to validate what works — it is saying we should not confuse MVP prototypes with a business-ready model.)
- Defensible AI business models are built on data. It is borderline impossible to have a model architecture so state-of-the-art that nothing will surpass its capabilities (on similar data inputs). It is comparatively easy to curate data sources and infrastructure that present a sufficiently high barrier to entry to prevent new competitors from entering your market with a meaningfully competitive product.
- The cost of good foundations are defrayed across all products and end-users.
- Defraying across many products makes profitable large data investments that no single product would justify, but have excellent ROI on a cohort of products. Disciplined planning should identify these opportunities.
- Defraying across many users makes profitable large data investments that individual customers would not make themselves.
Accepting the above advice as correct, there are significant conclusions about the future of practical AI in business. The economies of scale in specialised data collection suggest that AI SaaS providing services to industry sectors is likely to develop superior returns over individual companies in-housing AI talent save for the largest companies (analogies could be drawn to providers like ARM in chip design). There is also a huge unrealised potential for serial investors to bring data expertise and curated resources into SMEs.
summary
Data science creates value when it enables better decisions. The process of finding the right data and understanding it well enough to make those decisions, or build AI tools to continually produce the right decision, requires building upon a hierarchy of capabilities.
That hierarchy is expensive to build (but bad decisions built on a poor/nonexistent hierarchy are even more expensive), so businesses should tightly control investments in that capability to target projects with the best return.
Considering a product suite and the interdependent roots of the mountain range they require lets you better prioritize projects and where to invest in reusable data + infrastructure.
If you have any questions about practical AI, feel free to reach out to me or the Actifai team.
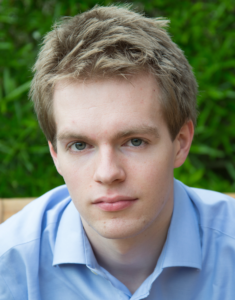
Jonathan Burley
Head of Data Science - Actifai
03.24.2023